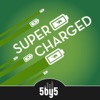
191: Replika: the Most Human Approach to Artificial Intelligence
Supercharged
English - March 19, 2018 00:00 - 1 hour - 56.1 MB - ★★★★ - 23 ratingsTechnology Education How To adam dachis tommy honton lifehacker productivity science psychology how-to life hack lifestyle Homepage Download Apple Podcasts Google Podcasts Overcast Castro Pocket Casts RSS feed
This week Tommy and Adam chat with Eugenia Kuyda, founder of Replika, a chat bot unlike pretty much any other as it is designed to be your friend—and actually succeeds.
We've discussed Replika on Supercharged several times and have been looking forward to this interview. If you haven't tried Replika yet, go do it! It's free to use for both Android and iOS and, with the recent open-sourcing of Replika's code, you can even build your own!
Got a question/comment/recommendation/etc. for Supercharged? Visit http://awkwardhuman.com/supercharged to send it in!
This week Tommy and Adam chat with Eugenia Kuyda, founder of Replika, a chat bot unlike pretty much any other as it is designed to be your friend—and actually succeeds.
We've discussed Replika on Supercharged several times and have been looking forward to this interview. If you haven't tried Replika yet, go do it! It's free to use for both Android and iOS and, with the recent open-sourcing of Replika's code, you can even build your own!
Got a question/comment/recommendation/etc. for Supercharged? Visit http://awkwardhuman.com/supercharged to send it in!
Links for this episode:
ReplikaI don't think we need to explain what this link is. :) Go download Replika!
CakeChat
Build your own Replika-esque chat bot! With the code open-sourced, complete with a pre-trained model, you can be up and running very quickly. You can, of course, train the model on your own data set and make adjustments. If you have development skills you can do this, even if you know very little about machine learning.
MILABOT: A Deep Reinforcement Learning Chatbot
Cornell University: "We present MILABOT: a deep reinforcement learning chatbot developed by the Montreal Institute for Learning Algorithms (MILA) for the Amazon Alexa Prize competition. MILABOT is capable of conversing with humans on popular small talk topics through both speech and text. The system consists of an ensemble of natural language generation and retrieval models, including template-based models, bag-of-words models, sequence-to-sequence neural network and latent variable neural network models. By applying reinforcement learning to crowdsourced data and real-world user interactions, the system has been trained to select an appropriate response from the models in its ensemble. The system has been evaluated through A/B testing with real-world users, where it performed significantly better than many competing systems. Due to its machine learning architecture, the system is likely to improve with additional data."