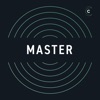
Generative models: exploration to deployment (Practical AI #240)
Changelog Master Feed
English - October 03, 2023 19:00 - 49 minutes - 45.1 MB - ★★★★ - 28 ratingsTechnology Education How To changelog open source oss software development developer hacker Homepage Download Apple Podcasts Google Podcasts Overcast Castro Pocket Casts RSS feed
What is the model lifecycle like for experimenting with and then deploying generative AI models? Although there are some similarities, this lifecycle differs somewhat from previous data science practices in that models are typically not trained from scratch (or even fine-tuned). Chris and Daniel give a high level overview in this effort and discuss model optimization and serving.
What is the model lifecycle like for experimenting with and then deploying generative AI models? Although there are some similarities, this lifecycle differs somewhat from previous data science practices in that models are typically not trained from scratch (or even fine-tuned). Chris and Daniel give a high level overview in this effort and discuss model optimization and serving.
Changelog++ members save 2 minutes on this episode because they made the ads disappear. Join today!
Sponsors:
Neo4j – NODES 2023 is coming in October!
Fastly – Our bandwidth partner. Fastly powers fast, secure, and scalable digital experiences. Move beyond your content delivery network to their powerful edge cloud platform. Learn more at fastly.com
Fly.io – The home of Changelog.com — Deploy your apps and databases close to your users. In minutes you can run your Ruby, Go, Node, Deno, Python, or Elixir app (and databases!) all over the world. No ops required. Learn more at fly.io/changelog and check out the speedrun in their docs.
Featuring:
Chris Benson – Twitter, GitHub, LinkedIn, WebsiteDaniel Whitenack – Twitter, GitHub, Website
Show Notes:
BigDL
Article: Making LLMs even more accessible with bitsandbytes, 4-bit quantization and QLoRA
Previous episode: Running large models on CPUs
Baseten’s Truss
Seldon
Hugging Face’s TGI
Intel Gaudi 2
Intel TDX
Something missing or broken? PRs welcome!