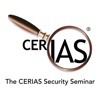
Neil Gong, Secure Federated Learning
CERIAS Weekly Security Seminar - Purdue University
English - June 09, 2021 17:30 - 1 hour - 410 MB Video - ★★★★ - 6 ratingsTechnology Education Courses infosec security video seminar cerias purdue information sfs research education Homepage Download Apple Podcasts Google Podcasts Overcast Castro Pocket Casts RSS feed
Federated learning is an emerging machine learning paradigm to enable many clients (e.g., smartphones, IoT devices, and edge devices) to collaboratively learn a model, with help of a server, without sharing their raw local data. Due to its communication efficiency and potential promise of protecting private or proprietary user data, and in light of emerging privacy regulations such as GDPR, federated learning has become a central playground for innovation. However, due to its distributed nature, federated learning is vulnerable to malicious clients. In this talk, we will discuss local model poisoning attacks to federated learning, in which malicious clients send carefully crafted local models or their updates to the server to corrupt the global model. Moreover, we will discuss our work on building federated learning methods that are secure against a bounded number of malicious clients. About the speaker: Neil Gong is an Assistant Professor in the Department of Electrical and Computer Engineering and Department of Computer Science (secondary appointment) at Duke University. He is broadly interested in cybersecurity with a recent focus on the intersections between security, privacy, and machine learning. He received a B.E. from the University of Science and Technology of China (USTC) in 2010 and a Ph.D in Computer Science from the University of California at Berkeley in 2015. He has received an NSF CAREER Award, an Army Research Office (ARO) Young Investigator Award, Rising Star Award from the Association of Chinese Scholars in Computing, an IBM Faculty Award, and multiple best paper or best paper honorable mention awards.