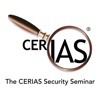
Michael Clark, "From Machine Learning Threats to Machine Learning Protection Requirements"
CERIAS Weekly Security Seminar - Purdue University
English - October 07, 2020 20:30 - 393 MB Video - ★★★★ - 6 ratingsTechnology Education Courses infosec security video seminar cerias purdue information sfs research education Homepage Download Apple Podcasts Google Podcasts Overcast Castro Pocket Casts RSS feed
Researchers from academia and industry have
identifiedinteresting threat vectors against machine learning
systems. These threatsexploit intrinsic vulnerabilities in the
system, or vulnerabilities that arisenaturally from how the system
works rather than being the result of a specificimplementation
flaw. In this talk, I present recent results in threats tomachine
learning systems from academia and industry, including some of our
ownresearch at Riverside Research. Knowing about these threats is
only half thebattle, however. We must determine how to transition
both the understandinggained by developing attacks and specific
defenses into practice to ensure thesecurity of fielded systems. In
this talk I leverage my experience working onstandards committees
to present an approach for leveraging machine learningprotection
requirements on systems that use machine learning.