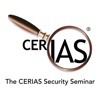
Gary McGraw, "Security Engineering for Machine Learning"
CERIAS Weekly Security Seminar - Purdue University
English - May 26, 2021 17:30 - 1 MB Video - ★★★★ - 6 ratingsTechnology Education Courses infosec security video seminar cerias purdue information sfs research education Homepage Download Apple Podcasts Google Podcasts Overcast Castro Pocket Casts RSS feed
Machine Learning appears to have made impressive progress on
many tasks including image classification, machine translation,
autonomous vehicle control, playing complex games including chess,
Go, and Atari video games, and more. This has led to much
breathless popular press coverage of Artificial Intelligence, and
has elevated deep learning to an almost magical status in the eyes
of the public. ML, especially of the deep learning sort, is not
magic, however. ML has become so popular that its application,
though often poorly understood and partially motivated by hype, is
exploding. In my view, this is not necessarily a good thing. I am
concerned with the systematic risk invoked by adopting ML in a
haphazard fashion. Our research at the Berryville Institute of
Machine Learning (BIIML) is focused on understanding and
categorizing security engineering risks introduced by ML at the
design level. Though the idea of addressing security risk in ML is
not a new one, most previous work has focused on either particular
attacks against running ML systems (a kind of dynamic analysis) or
on operational security issues surrounding ML. This talk focuses on
the results of an architectural risk analysis (sometimes called a
threat model) of ML systems in general. A list of the top five (of
78 known) ML security risks will be presented.