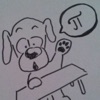
Typical Decoding for Natural Language Generation (Get more human-like outputs from language models!)
Yannic Kilcher Videos (Audio Only)
English - March 28, 2022 14:06 - 48 minutes - 45.3 MB - ★★★★★ - 1 ratingTechnology Homepage Download Apple Podcasts Google Podcasts Overcast Castro Pocket Casts RSS feed
#deeplearning #nlp #sampling
Modern language models like T5 or GPT-3 achieve remarkably low perplexities on both training and validation data, yet when sampling from their output distributions, the generated text often seems dull and uninteresting. Various workarounds have been proposed, such as top-k sampling and nucleus sampling, but while these manage to somewhat improve the generated samples, they are hacky and unfounded. This paper introduces typical sampling, a new decoding method that is principled, effective, and can be implemented efficiently. Typical sampling turns away from sampling purely based on likelihood and explicitly finds a trade-off between generating high-probability samples and generating high-information samples. The paper connects typical sampling to psycholinguistic theories on human speech generation, and shows experimentally that typical sampling achieves much more diverse and interesting results than any of the current methods.
Sponsor: Fully Connected by Weights & Biases
https://wandb.ai/fully-connected
OUTLINE:
0:00 - Intro
1:50 - Sponsor: Fully Connected by Weights & Biases
4:10 - Paper Overview
7:40 - What's the problem with sampling?
11:45 - Beam Search: The good and the bad
14:10 - Top-k and Nucleus Sampling
16:20 - Why the most likely things might not be the best
21:30 - The expected information content of the next word
25:00 - How to trade off information and likelihood
31:25 - Connections to information theory and psycholinguistics
36:40 - Introducing Typical Sampling
43:00 - Experimental Evaluation
44:40 - My thoughts on this paper
Paper: https://arxiv.org/abs/2202.00666
Code: https://github.com/cimeister/typical-...
Abstract:
Despite achieving incredibly low perplexities on myriad natural language corpora, today's language models still often underperform when used to generate text. This dichotomy has puzzled the language generation community for the last few years. In this work, we posit that the abstraction of natural language as a communication channel (à la Shannon, 1948) can provide new insights into the behaviors of probabilistic language generators, e.g., why high-probability texts can be dull or repetitive. Humans use language as a means of communicating information, and do so in a simultaneously efficient and error-minimizing manner; they choose each word in a string with this (perhaps subconscious) goal in mind. We propose that generation from probabilistic models should mimic this behavior. Rather than always choosing words from the high-probability region of the distribution--which have a low Shannon information content--we sample from the set of words with information content close to the conditional entropy of our model, i.e., close to the expected information content. This decision criterion can be realized through a simple and efficient implementation, which we call typical sampling. Automatic and human evaluations show that, in comparison to nucleus and top-k sampling, typical sampling offers competitive performance in terms of quality while consistently reducing the number of degenerate repetitions.
Authors: Clara Meister, Tiago Pimentel, Gian Wiher, Ryan Cotterell
Links:
Merch: store.ykilcher.com
TabNine Code Completion (Referral): http://bit.ly/tabnine-yannick
YouTube: https://www.youtube.com/c/yannickilcher
Twitter: https://twitter.com/ykilcher
Discord: https://discord.gg/4H8xxDF
BitChute: https://www.bitchute.com/channel/yann...
LinkedIn: https://www.linkedin.com/in/ykilcher
BiliBili: https://space.bilibili.com/2017636191
If you want to support me, the best thing to do is to share out the content :)