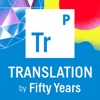
Low-N Protein Engineering with Surge Biswas
Translation
English - October 06, 2020 05:16 - 29 minutes - 27.1 MB - ★★★★★ - 10 ratingsLife Sciences Science Technology science 50y fifty years research translation biology phd synbio spinout impact Homepage Download Apple Podcasts Google Podcasts Overcast Castro Pocket Casts RSS feed
Protein engineering has been dominated by two opposing paradigms; directed evolution, a massive screening technique, and rational design, a completely computational approach. Surge has fused these two paradigms by developing a machine learning technique that discovers an optimal protein design by training on a low number of engineered proteins. Here, Surge discusses how this hybrid method works, how it enabled the creation of better fluorophores and enzymes, and what this method will unlock next.
About the Author
Surge performed this work as a graduate student at Harvard in the lab of Professor George Church. George is one of the founding fathers of synthetic biology and the lab is known for developing high throughput methods to design, build, and test bioengineered parts.As CEO and co-founder of Nabla Bio, Surge is now focused on pointing the algorithms and methods developed in his academic work toward building proteins that can improve human health or protect the environment.Key Takeaways
Methods from natural language processing algorithms (like Siri or Alexa) are adapted to understand how nature builds proteins.These machine learning algorithms distill fundamental structural, as well as evolutionary and and biophysical properties about proteins.Fusing these models with real world data enables us to make proteins with improved or novel functionality.By checking how a few mutations (low-n) affect the function of a protein, Surge evolved proteins in a computer to make better fluorescent proteins and enzymes.These models know a lot about proteins in general and can therefore be applied to a wide variety of tasks that improve human health and protect the environment.Translation
This methodology dramatically reduces the time, cost, and labor of evolving proteins, making it a perfect tool to create commodity proteins.Based on this technology, Surge co-founded Nabla Bio whose goal is to engineer supernatural proteins that enable biology to solve the world's biggest problems.First Author: Surojit “Surge” Biswas
Paper: Low-N protein engineering with data-efficient deep learning. bioRxiV, 2020.
Follow Fifty Years on Twitter!
If you’re an author of an upcoming paper in bio or know of any interesting papers dropping soon and want to hear from the authors, drop us an email at translation [AT] fifty [DOT] vc.