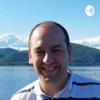
Do you trust in pretrained Deep Learning models?
Thales Sehn Körting
English - November 19, 2019 22:42 - 6 minutes - 6.28 MBEarth Sciences Science Homepage Download Apple Podcasts Google Podcasts Overcast Castro Pocket Casts RSS feed
Several authors rely on transfer learning from pretrained models, arguing that using well-known datasets, which are available on the internet (e.g. ImageNet) their model will be able to handle a specific problem with a reduced training step.
In Remote Sensing this perspective is also becoming a trend when using Deep Learning techniques to classify Remote Sensing datasets.
In my opinion, the datasets used for pretrain are very different from Remote Sensing targets, mainly in two aspects:
spatial resolution: a sensor can be ultra high spatial resolution (50cm for example) or very low resolution (2km for a single pixel), and the edges in all these images are different
spectral resolution: the datasets found on the internet are composed by color pictures, obtained mainly by phone cameras, which are composed by 3 channels (red, green and blue). In Remote Sensing we can have several spectral channels, such as yellow or red-edge bands (available in WorldView-2), or infra-red channels, available in most of the satellites. How to train a model using 3 bands, when in reality you can have at least 5 bands with so different information?
If you agree, or if you do not agree, please give some feedback and let's learn together.
Follow my podcast: http://anchor.fm/tkorting
Subscribe to my YouTube channel: http://youtube.com/tkorting
The intro and the final sounds were recorded at my home, using an old clock that belonged to my grandmother.
Thanks for listening