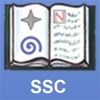
Highlights From The Comments On Motivated Reasoning And Reinforcement Learning
Astral Codex Ten Podcast
English - February 13, 2022 14:28 - 23 minutes - 27 MB - ★★★★★ - 63 ratingsTechnology Homepage Download Apple Podcasts Google Podcasts Overcast Castro Pocket Casts RSS feed
https://astralcodexten.substack.com/p/highlights-from-the-comments-on-motivated
I. Comments From People Who Actually Know What They’re Talking About
Gabriel writes:
The brain trains on magnitude and acts on sign.
That is to say, there are two different kinds of "module" that are relevant to this problem as you described, but they're not RL and other; they're both other. The learning parts are not precisely speaking reinforcement learning, at least not by the algorithm you described. They're learning the whole map of value, like a topographic map. Then the acting parts find themselves on the map and figure out which way leads upward toward better outcomes.
More precisely then: The brain learns to predict value and acts on the gradient of predicted value.
The learning parts are trying to find both opportunities and threats, but not unimportant mundane static facts. This is why, for example, people are very good at remembering and obsessing over intensely negative events that happened to them -- which they would not be able to do in the RL model the post describes! We're also OK at remembering intensely positive events that happened to us. But ordinary observations of no particular value mostly make no lasting impression. You could test this by a series of 3 experiments, in each of which you have a screen flash several random emoji on screen, and each time a specific emoji is shown to the subject, you either (A) penalize the subject such as with a shock, or (B) reward the subject such as with sweet liquid when they're thirsty, or (C) give the subject a stimulus that has no significant magnitude, whether positive or negative, such as changing the pitch of a quiet ongoing buzz that they were not told was relevant. I'd expect subjects in both conditions A and B to reliably identify the key emoji, whereas I'd expect quite a few subjects in condition C to miss it.
By learning associates with a degree of value, whether positive or negative, it's possible to then act on the gradient in pursuit of whatever available option has highest value. This works reliably and means we can not only avoid hungry lions and seek nice ripe bananas, but we also do
https://astralcodexten.substack.com/p/highlights-from-the-comments-on-motivated
I. Comments From People Who Actually Know What They’re Talking About
Gabriel writes:
The brain trains on magnitude and acts on sign.
That is to say, there are two different kinds of "module" that are relevant to this problem as you described, but they're not RL and other; they're both other. The learning parts are not precisely speaking reinforcement learning, at least not by the algorithm you described. They're learning the whole map of value, like a topographic map. Then the acting parts find themselves on the map and figure out which way leads upward toward better outcomes.
More precisely then: The brain learns to predict value and acts on the gradient of predicted value.
The learning parts are trying to find both opportunities and threats, but not unimportant mundane static facts. This is why, for example, people are very good at remembering and obsessing over intensely negative events that happened to them -- which they would not be able to do in the RL model the post describes! We're also OK at remembering intensely positive events that happened to us. But ordinary observations of no particular value mostly make no lasting impression. You could test this by a series of 3 experiments, in each of which you have a screen flash several random emoji on screen, and each time a specific emoji is shown to the subject, you either (A) penalize the subject such as with a shock, or (B) reward the subject such as with sweet liquid when they're thirsty, or (C) give the subject a stimulus that has no significant magnitude, whether positive or negative, such as changing the pitch of a quiet ongoing buzz that they were not told was relevant. I'd expect subjects in both conditions A and B to reliably identify the key emoji, whereas I'd expect quite a few subjects in condition C to miss it.
By learning associates with a degree of value, whether positive or negative, it's possible to then act on the gradient in pursuit of whatever available option has highest value. This works reliably and means we can not only avoid hungry lions and seek nice ripe bananas, but we also do