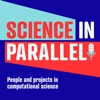
Season One, Episode Two -- Artificial Intelligence and Climate Change
Science in Parallel
English - July 15, 2021 21:17 - 34 minutes - 46.8 MB - ★★★★★ - 3 ratingsMathematics Science Technology energy hpc supercomputer artificialintelligence computationalscience computerscience computing csgf doecsgf doenationallabs Homepage Download Apple Podcasts Google Podcasts Overcast Castro Pocket Casts RSS feed
One of today’s hottest areas of computational research could help build better solutions for one of global society’s steepest challenges. Three early career computational scientists talk about AI’s potential for understanding and predicting climate shifts, supporting strategies for incorporating renewable energy, and engineering other approaches that reduce carbon emissions. They also describe how AI can be misused or can perpetuate existing biases.
Working at this important research interface requires broad knowledge in areas such as climate science, public policy and engineering coupled with computational science and mathematics expertise. These early career researchers talk about their approaches to bridging this gap and offer their advice on how to become a scientific integrator.
You’ll meet:
Priya Donti is a Ph.D. student at Carnegie Mellon University, pursuing a dual degree in public policy and computer science, and a 4th year DOE CSGF recipient. She is also a co-founder and chair of the volunteer organization, Climate Change AI, which provides resources and a community for researchers interested in applying artificial intelligence to climate challenges. Priya was named to MIT Technology Review’s 2021 list of Innovators Under 35. Read more about Priya and her work in the 2021 issue of DEIXIS.
Kelly Kochanski completed a Ph.D. in geological sciences at the University of Colorado, Boulder in 2020 and works as a senior data scientist in climate analytics at McKinsey & Company. Kelly was a DOE CSGF recipient from 2016 to 2020, and her graduate research was featured in the 2020 issue of DEIXIS. She also is profiled in the 2021 issue as one of this year’s recipients of the Frederick A. Howes Scholar Award.
Ben Toms also finished his Ph.D. last year at Colorado State University studying atmospheric science and is a 4th year DOE CSGF recipient. He has founded a company, Intersphere, that provides weather and climate forecasts up to a decade into the future.
From the episode:
Kelly and Priya contributed to the review article: Tackling Climate Change with Machine Learning, which was published on the arXiv preprint server in 2019. In the discussion about interpretable AI, Priya mentioned an article by Cynthia Rudin: Stop explaining black box machine learning models for high stakes decisions and use interpretable models instead. Ben mentioned Vulcan’s work to build faster climate change models.