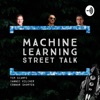
New 50% ARC result and current winners interviewed
Machine Learning Street Talk (MLST)
English - June 18, 2024 21:57 - 2 hours - 185 MBTechnology Homepage Download Google Podcasts Overcast Castro Pocket Casts RSS feed
The ARC Challenge, created by Francois Chollet, tests how well AI systems can generalize from a few examples in a grid-based intelligence test. We interview the current winners of the ARC Challenge—Jack Cole, Mohammed Osman and their collaborator Michael Hodel. They discuss how they tackled the ARC (Abstraction and Reasoning Corpus) Challenge using language models and neural networks. We also discuss the new "50%" approach announced today from Redwood Research.
Jack and Mohammed explain their approach, which involves fine-tuning a language model on a large, specially-generated dataset and then doing additional fine-tuning at test-time, a technique known in this context as "active inference". They use various strategies to represent the data for the language model and believe that with further improvements, the accuracy could reach above 50%. Michael talks about his work on generating new ARC-like tasks to help train the models.
Tim and the guests also debate whether their methods stay true to the spirit of measuring intelligence as intended by ARC's creator Francois Chollet. Despite some concerns, they agree that their solutions are promising and adaptable for other similar problems. The conversation wraps up with the guests encouraging others to explore the ARC tasks and share their creative solutions.
Jack Cole:
https://x.com/Jcole75Cole
https://lab42.global/community-interview-jack-cole/
Mohamed Osman:
Mohamed is looking to do a PhD in AI/ML, can you help him?
Email: [email protected]
https://www.linkedin.com/in/mohamedosman1905/
Michael Hodel:
https://arxiv.org/pdf/2404.07353v1
https://www.linkedin.com/in/michael-hodel/
https://x.com/bayesilicon
https://github.com/michaelhodel
Getting 50% (SoTA) on ARC-AGI with GPT-4o - Ryan Greenblatt
https://redwoodresearch.substack.com/p/getting-50-sota-on-arc-agi-with-gpt