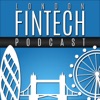
LFP176 – Sci-Fi Creeps Ever Nearer – Automated Detection of Emotion In Voice Audio w/Rana Gujral Behavioral Signals
London Fintech Podcast
English - April 08, 2021 04:05 - 44 minutes - 41.1 MB - ★★★★★ - 8 ratingsBusiness Technology Homepage Download Apple Podcasts Google Podcasts Overcast Castro Pocket Casts RSS feed
Behavioral Signals aims to “turn your conversational data into actionable insights for your business” via the automated cross-cultural detection of emotions in the audio of conversations. This is a super-new front in machine language recognition and usage one which has to date been rather simplistically approached but one which as we all know every day […]
Behavioral Signals aims to “turn your conversational data into actionable insights for your business” via the automated cross-cultural detection of emotions in the audio of conversations. This is a super-new front in machine language recognition and usage one which has to date been rather simplistically approached but one which as we all know every day is essential to real human interaction – witness all the confusion that for example emails can cause which would not occur if we saw people’s body language and heard the tone of what they were saying as well as simply the content in Times New Roman. Indeed it is this deficiency of the latter which led to the invention of emoticons to crudely add back some disambiguation.
Rana Gujral, CEO of Behavioral Signals, is a super-experienced veteran entrepreneur and CEO in Silicon Valley and in this episode he draws the curtain back on if not the final frontier then certainly a might important new one of man-machine interfacing.
Alexa and Siri can do an amazing job today compared to their predecessors a decade or two back, yet neither of them have any sense that they are dealing with a human being – they simply detect “words in Times New Roman” as it were and have no concept that the person asking is a human being for whom words are at times a small part of the bandwidth.
In this show Rana covers one Case Study of a usage of this technology in FS which ably demonstrated that when one is dealing with real technological innovation the use case innovation is itself also truly radical and requires large amounts of initiative and imagination to think beyond the obvious – after all if people already detect emotion how could a computer supplement that?
Topics discussed include:
mountain biking in San Francisco during lockdown
Rana’s impressive career journey and interesting product journeys – a great reminder that the West Coast of the States has really led in deep innovation for decades
California, tech, Texas and Elon Musk
the challenges of extreme innovation
huge unexplored potential use case domains
how computer-voice innovation has developed in recent decades
affect is relatively new given the tall order of getting computers to understand multiple speakers in multiple languages in the first place
NLP and NLU
in this quest tone of voice has historically been discarded despite embedding very valuable (sic) information
emotions as ranging from visceral through to mental influenced and as often the most important undertone of a conversation – you cant understand or even hold for long a conversation without modelling the emotional state of the person you are speaking to
the necessity as well as opportunity of being able to process this layer of the information
parsing voice into words is a challenge but schematically simple
how does one parse audio for emotion?
Behavioral Signals exclusively focus on tonality – pitch and tonal variance – in order to extract the underlying sentiment/emotion – “it’s not just what you are saying it but how you are saying it” that conveys information
Psychologists have produced models of how emotion is reflected in voice – factor analysis
simplest model is a two-factor model: balance (how +ve/-ve) and arousal – and one can plot emotions on that two-D chart as a starting point for measuring core affect
the heart of BS approach is along these lines to derive core affect
the importance of having a whole pipeline of components that interact – cf a car engine is not a thing but a connection of things that together produce the desired result
the various buckets that this process can derive from an audio of speech
prior established products are based purely on linguistic analysis – eg use of a word “amazing” would lead to a positive assessment with no way of measuring eg sarcasm – hence affect detection is a huge leap forwards from that
cross-cultural detection of affect/emotion – is this a huge challenge or is emotion more basic than language (after all babies around the world communicate core emotions in the same way from day one, even if social training will impact the outward display of inward emotion over time)
how this is approached and why it works (cf GSR in lie detectors)
Case Study of their “AI mediated Conversations” – AIMC – product to match appropriate call centre staff with debt collection conversations based on the emotional patterns in voice
the amazing increase in debt collected of 10-15% as a result of matching
not only do collections go up but the client satisfaction is greater too – most people seek to sort out their debt situation
B.S. was founded in 2016 out of Uni Souther California
HQ in LA, small team in SF and larger team in Athens, Greece
active in Europe as well as US
focused on banks and collection conglomerates right now
And much more more
Share and include!