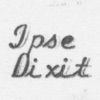
Aaron Roth & Michael Kearns on Ethical Algorithms
Ipse Dixit
English - March 03, 2020 07:32 - 1 hour - 27.8 MB - ★★★★★ - 98 ratingsNews Society & Culture Philosophy law legal scholarship jurisprudence scholarship academia Homepage Download Apple Podcasts Google Podcasts Overcast Castro Pocket Casts RSS feed
In this episode, Aaron Roth and Michael Kearns, both professors of computer science at the University of Pennsylvania, discuss their new book, The Ethical Algorithm (Oxford U. Press 2020). This wide-ranging interview covers issues related to privacy, fairness, and explainable algorithms. The interview has three parts. First, Aaron and Michael explain the goal, process, and limits of differential private data. Second, they discuss various definitions of fairness in algorithmic decision-making and point out that simultaneously maximizing fairness along several dimensions may be impossible to achieve. Finally, they briefly discuss how an inscrutable, “black box” algorithm can be made to produce explainable decisions in specific contexts. Most interestingly, the authors provide several examples of how the process of translating laws into specific directions that machine learning models can follow may demonstrate that our laws are asking for the impossible. For example, it may be impossible to create a credit-underwriting algorithm that is “fair” to both women and black borrowers at the same time despite what the Equal Credit Opportunity Act purports to require. Roth and Kearns are both on Twitter at @Aaroth and @mkearnsupenn, respectively. This episode was hosted by Matthew Bruckner, an associate professor of law at Howard University School of Law. He is on Twitter at @Prof_Bruckner.
Hosted on Acast. See acast.com/privacy for more information.