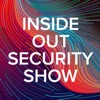
Attorney and Data Scientist Bennett Borden: Find Insider Threats (Part 2)
State of Cybercrime
English - September 27, 2016 14:24 - 21 minutes - 19.7 MB - ★★★★★ - 48 ratingsTechnology Business Homepage Download Apple Podcasts Google Podcasts Overcast Castro Pocket Casts RSS feed
In this second podcast, Bennett continues where he left off last time. Borden describes his work on developing algorithms to find insider threats based on analyzing content and metadata.
Transcript
Cindy: Hi, everyone. Welcome to our Inside Out Security Podcast. Andy and I are very excited to have Bennett Borden. He plays many roles. He is an attorney, a data scientist, and also a partner at Drinker Biddle in Washington D.C. So welcome, Bennett.
Andy: Thanks, Cindy. And again welcome, Bennett. Thank you for joining this call. So we're really excited to have you, and mostly because you have this unusual background that bridges law and data analysis. You've also written some really interesting articles on the subject of applying data science to e-discovery. I'm wondering for our non-lawyer readers of the blog, can you tell us what discovery is and how it has led to the use of big data techniques?
Bennett: Sure, absolutely. And Andy and Cindy, thanks for having me. So discovery is a process in litigation. And it's a process when two or more parties get into litigation. These rules about discovery require the parties to trade information about whatever the case is about.
So if you think of a patent infringement case or a breach of contract case, the two parties ‘serve discovery’—that’s what it's called--on each other. This is basically a game of Go Fish. And one side says, "Give me all your documents about the formation of the contract," and then the other side has to go and find all those documents.
As you can imagine, in the information age, that could be anything!
You've got to go find all the emails about that and all the documents like Word or PowerPoint. Depending on the case, it could be things like server logs or financial or HR data. It becomes quite the hunt in this modern age.
Varonis: Right. So it has become e-discovery or electronic discovery.
Bennett: That's exactly right.
Varonis: The problem is finding relevant documents. And this problem of finding relevancy and how to decide whether a document is relevant would seem to lead to some ideas in data science.
Bennett: Yes, and that's what's been really great about the advent of the information age and big data analytics in the last few years. Discovery has been around since the 1960's, but it was initially a paper endeavor. You had to go to file cabinets and file rooms and you'd find stuff, and copy it, and hand it over.
But as we’ve gotten into computerized systems and databases and especially email, it's become really quite burdensome. Millions of dollars are spent trying to find and locate these documents. It began as an issue of search technology, having to search these different repositories, document management systems and file servers and email servers.
Then as data analytics came online, we have these advanced machine learning search capabilities. As I find something that I'm looking for, it's basically a “more like this” search, and analytical tools can help us understand the characteristics of what they call responsive documents and help us find more like that. It's greatly increased the efficiency of the discovery process.
Varonis: Right. So it seems like some of these ideas of using data science started with e-discovery, but it has branched out from there. And I know you've written about how data analytics was used, for example, in other legal transactions like a mergers and acquisition case that you wrote about. Can you tell us more about how it's expanding from just e-discovery?
Bennett: Yeah. This is really what's one of the most interesting parts of data science and its convergence in the legal sphere, because if you think about it, a lawyer's most fundamental product is really information.
As a litigator, as a corporate lawyer, what we're trying to figure out is what happened and why: sometimes it’s whose fault it is or even trying to understand the value of a transaction or the value of a company, or the risk that's associated with certain kinds of securities transactions. All of that is based on information. The easier it is and the more accurately and quickly you can get at certainty of information, the better legal product you have.
We started playing with these techniques. It’s the same techniques that were helping us find information that was relevant to a case, and tried to apply these to different settings.
One of the most obvious is investigation settings, like a regulatory investigation or even an internal investigation. It's the same kind of principle, you're looking for electronic evidence of what happened. And that kind of pushed us into some interesting other areas.
If you think about how a merger or acquisition happens, company A wants to buy company B, and so company A asks a bunch of questions — what they call due diligence. They want to know what your assets and liabilities are, what risks you might face, what are your uncollectible accounts, and, say, do you have any kind of environmental risk or litigation going on.
The information provided by the target company is used to get an understanding of the value of the target, and that's what determines the purchase price. The more certain that information is or that value is, the fairer the price. When you start getting fluctuations in price, it really reflects the amount of uncertainty over the value of the company.
Often when these companies trade information, they don't have a clear picture of the other side. We started using these electronic discovery and big data techniques in the due diligence process to get clearer information.
Varonis: Right. As I recall from the case, the company was sold, and then as part of verifying the company's disclosures about what they were doing were accurate, you actually went and looked at the file system.
Bennett: Yeah. And this is what's interesting! When we were talking to our M&A lawyers, as one of the endeavors, we were going around to different practice groups in the firm, and we were saying, "Look, I have this skill set where I can get you information. And so how would that be valuable to you?"
One of the lawyers I was talking to, the head of the M&A group, said, ‘Look, our biggest problem is that we don't have about what they're telling us and how accurate it is.’
Every M&A transaction has a provision, which they call an indemnification provision, that basically says, ‘You're going to tell me about your company, and then I'm going to give you some money for the company, but if I find out that what you told me was not accurate, then to the extent it wasn't accurate, I get to adjust my purchase price after.’ In other words, I get a refund of whatever the differences in value are.
The problem with these indemnification provisions is that they are only open for like 30 or 60 days. Usually it's very hard to figure out whether the information is accurate within that very short period of time.
So in this particular case, our client, the purchaser, had some doubts about the veracity of some of the information coming out of the other side, but really couldn't prove one way or the other. Literally the day that the purchase closed, we owned all the information assets at the company we bought.
We swooped in and did a data analysis, looking at all the information they had given us, and then walked it back through time. How did they come up with these figures in their disclosures? What was the internal discussion going on with their internal people and their outside auditors?
We were able to show there was a pretty wide variance between what they told us and what is a reasonable basis for the valuation.
We got millions of dollars back on that purchase price, and we've been able to do that over and over again now because we are able to get at these answers much more quickly in electronic data.
Varonis: Right. Yeah. It's fascinating that you're able to find the relevant files, you connect them over a period of time, and then sort of walk it back as a way to learn that the disclosure was not quite right.
We're definitely on the same page about there's a lot of information on file systems, and data science can help pull it out!
Back in December, we heard you speak at the CDO Summit here in New York City, and you also mentioned a system that you helped develop that can spot insider threats during and even before the actual incident. And I think you said you analyzed both the actual content and meta-content or metadata. Can you talk a little bit more about that system?
Bennett: Sure. You know, this is one of the most intriguing things that I think we've done. And it sprang out of this understanding that electronic data inside of a company, and really anywhere, is really evidence of where someone has been at a certain point in time, and what they thought or did or purchased or communicated. So you can actually watch how decisions are made or how actions start to be undertaken.
All of us go through our everyday lives, especially at work, leaving trails behind of conversations with people, emails back and forth, and how we come to decisions. All of these things are now kept in this electronic record. We have this sociological record-- more than we've ever had as a species, really! If you know how to get at those facts and how to put them together, you can really find the answer to just about how anything came about.
I came out of the intelligence community before going to law school, and so figuring out what happened and why has been my background for my entire career.
What we figured out in data science is we are pretty good at being able to predict what people are going to do as consumers. For example, this is why your Amazon suggestions, your gift basket, or your Netflix suggestions on movies, or the coupons they spit out at the local pharmacy are based on predictions of what you’re going to do.
I thought if we can predict what someone's going to like or what someone's going to buy, surely I can predict if someone's going to do something wrong, because just like there's patterns in all human conduct, there's patterns in misconduct as well.
So we tested this. We took a number of data sets that we had basically found in a discovery process-- a litigation or a regulatory investigation that was about corporate misconduct, something like financial statement fraud-- and all of these documents had already been analyzed by teams of lawyers to figure out which ones of those were relevant to the underlying misconduct.
I had a target variable that a document was or was not related to the underlying misconduct.
We built algorithmic models, predictive models, based on these underlying data, across all sorts of different kinds of misconduct, and it turned out that misconduct is actually highly predictable.
We worked with some of my colleagues back at the intelligence agencies, some folks at the FBI, and some social scientists who worked with the psychology of fraud and the psychology of wrongdoing, and developed this algorithmic model that had aspects of text mining--- words and phrases people used.
Some of it was based on social network analysis—looking at who was talking to who, and when, or strange patterns in communication, especially outside of work hours, people that don't normally talk to each other, or siphoning off communications outside of the network to personal email accounts.
A significant part of this was conducting sentiment analysis. It turns out that the sentiment analysis actually was a large proportion of the predictive algorithm. After putting all of these features together into a model, it was stunningly accurate that we could find patterns as it began to develop, that people were either engaging in some kind of misconduct, or a situation was ripe where such misconduct could occur.
Varonis: Right. There's some really interesting research about what they call precursors of insiders, and it sounds like you're spotting that in your algorithms. It also seems like you have a training set of data and you build it up, creating profile, a statistical profile.
Bennett: Yes, that's exactly right. We looked specifically at the temporal aspect of misconduct. Catching someone after they've done it or kind of after the horse is out of the barn, that's easier, but what's harder is can we actually see the misconduct coming?
So we built this model where we had a test set of data, some of which we used to build the model, and of course some of which we used to test it on, and we focused specifically on the behavior leading up to the misconduct. So could we catch it earlier?
And that's where it's really interesting to see the dynamics, the sociological dynamics of how a corporation works, and people's frustration level and feelings of acceptance and support--was there some kind of loyalty severancing event? It was really quite an interesting sociological effort.
Varonis: Absolutely, yeah. The researchers talk about trigger events that will push an insider over the line, so to speak. But we've also learned, as you suggested, that the insiders will actually try it out, they'll try to do some test runs to see how far they can get. And it sounds like your algorithms would then spot this. In other words, stop them before they actually do the act of copying or destruction or whatever it is.
Bennett: That's exactly it.
Varonis: Yeah. We call that user behavior analytics, or UBA. That's the industry term of it. So it sounds like you think that's the right approach. Not everyone follows that way of finding these behaviors, or catching insiders, I should say, but it sounds like behavior is something that you're very interested in spotting.
Bennett: It is. You know, it's fraught with very interesting issues. One of the things that I speak about quite often is the ethical use of data analytics. And there are certainly issues here. A lot of the triggering events or the triggers that come into misconduct situations have to do with people's personal lives, some kind of personal crisis or financial crisis, drug or alcohol dependencies, and a lot of it has to do with their interaction with their colleagues and superiors, stressful situations and feelings of ingratitude or not being recognized for their worth, and those are very personal things.
And one of the things that we tested this on as part of a graduate program that I was involved in at New York University is what could you tweak this algorithm to find? We actually did some test runs. Could you find all the Republicans? Could you find people of a particular political belief or such? And you can!
And it's very disconcerting that to realize that these kinds of algorithms can really find just about anything. So then the question becomes, "What's the right thing to do?"
What responsibility do we have as a company or especially a public company to monitor behavior and to monitor compliance, and yet not interfere with people's personal lives? It's a very interesting question that the law is really not settled on, and is something that we have to consider as data analysts.
Varonis: Yeah, that's very interesting. The question is, "When do you turn it on?" Should it be on all the time or do certain conditions justify it? So yeah, I absolutely agree that is an issue for companies.
I have one last question for you, and it has to do with, again, the insiders let's say who go bad, they're in a powerful position, they actually have created the content, they feel like they own it.
And these people are sometimes very hard to spot because they're the creators, they could be high level executives, they own the content, and sometimes hard to determine whether they've actually done something wrong.
They may be copying a lot of directories or files to a laptop, but that's just part of their job. So we are big believers in just keeping some basic audit trails on file activities, outside of any of the algorithms that we were talking about. So do you think that is just a minimal thing for companies to do?
Bennett: It is. It's interesting because it's why we built the algorithms to capture so many different kinds of behaviors so that one person could not hide their trail well enough. But there's just basic things the companies should do to understand where their most valuable information is and where it's going. There is very simple technology out there that allows us to understand where valuable information is being routed and where it goes to that are far away from these kind of advanced algorithms.
So it's common sense. In the Information Age a company's most valuable asset really is information. And so having what we call information governance principles, and so understanding and governing your information as you would any other asset is just good business.
Varonis: Right, absolutely agree.
So thank you so much, Bennett, on your insights today. Bennett, if people want to learn more about what you do and follow you on Twitter, do you have a handle or a website that you can share with everyone?
Bennett: Yes, thanks. My handle is @BennettBorden. And then most of my publications are on the firm's webpage at DrinkerBiddle.com under my profile. We write fairly often on this, and I would certainly welcome any thoughts from your listeners.