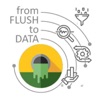
Episode 01 - Mechanistic Machine Learning
Flush to Data
English - July 10, 2020 15:00 - 1 hour - 66.1 MBNatural Sciences Science Mathematics Homepage Download Google Podcasts Overcast Castro Pocket Casts RSS feed
This is the first episode of the Flush to Data podcast. We start with a discussion on mechanistic modelling and machine learning and venture into models for emulation, uncertainty quantification, and data quality. Bonus material includes a discussion on aspects of current scientific practice, including the lack of hypothesis testing, the evaluation of novelty, and the challenges with a generalist approach.
Hosts: Jörg Rieckermann and Kris Villez
Guest: Juan Pablo Carbjal
Links:
* Juan Pablo's web page: https://sites.google.com/site/juanpicarbajal/
* Article relating Gaussian processes and Kalman filter: www.jstor.org/stable/2984861
* BBC podcast on Gauss: https://www.bbc.co.uk/programmes/b09gbnfj
* Using Lake Zurich as a heat sink: Unfortunately, we could not back-track the original source, despite considerable effort. If anyone of the listeners happens to know how to access the original source we would be grateful for a notice. The best we could find was documentation of related projects by Eawag: https://thermdis.eawag.ch/ and [1]. These show that ecological consequences have indeed been assessed in detail.
* Goodhart's law: https://en.wikipedia.org/wiki/Goodhart's_law
* An invitation to reproducible computational research: https://doi.org/10.1093/biostatistics/kxq028
* Science in the age of selfies: https://doi.org/10.1073/pnas.1609793113
References:
[1] Wüest, A. (2012). Potential zur Wärmeenergienutzung aus dem Zürichsee. Machbarkeit. Wärmeentzug (Heizen) und Einleitung von Kühlwasser. Kastanienbaum: Eawag. DORA-Link
Episode guide:
[0:00:00] Who is Juan Pablo Carbajal?
[0:03:10] Mechanistic modelling versus artificial intelligence
[0:07:08] Who is Juan Pablo Carbajal? (ctd.)
[0:09:26] Cross-fertilization between robotics and wastewater engineering
[0:15:05] Emulation: using models to approximate other models
[0:21:22] Incorporating common sense and prior knowledge into data-driven models
[0:31:31] Equivalence between Gaussian processes and Kalman filter
[0:33:50] Utility of emulation
[0:40:15] Utility of quantified uncertainty
[0:44:50] Intermezzo
[0:49:04] What can models say about data quality
[1:02:15] How to communicate about data quality?
[1:10:10] Preparing engineers for the future
[1:15:23] Thank you and goodbye!
Bonus material:
[1:16:40] Interpretable machine learning models
[1:22:33] Hypothesis testing
[1:26:14] Critical assessment of novelty
[1:30:50] Barriers to the generalist approach
[1:35:48] Thank you and goodbye!