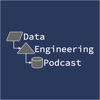
TimescaleDB: Fast And Scalable Timeseries with Ajay Kulkarni and Mike Freedman - Episode 18
Data Engineering Podcast
English - February 11, 2018 16:00 - 1 hour - 46.9 MB - ★★★★★ - 127 ratingsTechnology data engineering big data database data pipeline Homepage Download Apple Podcasts Google Podcasts Overcast Castro Pocket Casts RSS feed
Summary
As communications between machines become more commonplace the need to store the generated data in a time-oriented manner increases. The market for timeseries data stores has many contenders, but they are not all built to solve the same problems or to scale in the same manner. In this episode the founders of TimescaleDB, Ajay Kulkarni and Mike Freedman, discuss how Timescale was started, the problems that it solves, and how it works under the covers. They also explain how you can start using it in your infrastructure and their plans for the future.
Preamble
Hello and welcome to the Data Engineering Podcast, the show about modern data infrastructure
When you’re ready to launch your next project you’ll need somewhere to deploy it. Check out Linode at dataengineeringpodcast.com/linode and get a $20 credit to try out their fast and reliable Linux virtual servers for running your data pipelines or trying out the tools you hear about on the show.
Go to dataengineeringpodcast.com to subscribe to the show, sign up for the newsletter, read the show notes, and get in touch.
You can help support the show by checking out the Patreon page which is linked from the site.
To help other people find the show you can leave a review on iTunes, or Google Play Music, and tell your friends and co-workers
Your host is Tobias Macey and today I’m interviewing Ajay Kulkarni and Mike Freedman about Timescale DB, a scalable timeseries database built on top of PostGreSQL
Interview
Introduction
How did you get involved in the area of data management?
Can you start by explaining what Timescale is and how the project got started?
The landscape of time series databases is extensive and oftentimes difficult to navigate. How do you view your position in that market and what makes Timescale stand out from the other options?
In your blog post that explains the design decisions for how Timescale is implemented you call out the fact that the inserted data is largely append only which simplifies the index management. How does Timescale handle out of order timestamps, such as from infrequently connected sensors or mobile devices?
How is Timescale implemented and how has the internal architecture evolved since you first started working on it?
What impact has the 10.0 release of PostGreSQL had on the design of the project?
Is timescale compatible with systems such as Amazon RDS or Google Cloud SQL?
For someone who wants to start using Timescale what is involved in deploying and maintaining it?
What are the axes for scaling Timescale and what are the points where that scalability breaks down?
Are you aware of anyone who has deployed it on top of Citus for scaling horizontally across instances?
What has been the most challenging aspect of building and marketing Timescale?
When is Timescale the wrong tool to use for time series data?
One of the use cases that you call out on your website is for systems metrics and monitoring. How does Timescale fit into that ecosystem and can it be used along with tools such as Graphite or Prometheus?
What are some of the most interesting uses of Timescale that you have seen?
Which came first, Timescale the business or Timescale the database, and what is your strategy for ensuring that the open source project and the company around it both maintain their health?
What features or improvements do you have planned for future releases of Timescale?
Contact Info
Ajay
LinkedIn
@acoustik on Twitter
Timescale Blog
Mike
Website
LinkedIn
@michaelfreedman on Twitter
Timescale Blog
Timescale
Website
@timescaledb on Twitter
GitHub
Parting Question
From your perspective, what is the biggest gap in the tooling or technology for data management today?
Links
Timescale
PostGreSQL
Citus
Timescale Design Blog Post
MIT
NYU
Stanford
SDN
Princeton
Machine Data
Timeseries Data
List of Timeseries Databases
NoSQL
Online Transaction Processing (OLTP)
Object Relational Mapper (ORM)
Grafana
Tableau
Kafka
When Boring Is Awesome
PostGreSQL
RDS
Google Cloud SQL
Azure DB
Docker
Continuous Aggregates
Streaming Replication
PGPool II
Kubernetes
Docker Swarm
Citus Data
Website
Data Engineering Podcast Interview
Database Indexing
B-Tree Index
GIN Index
GIST Index
STE Energy
Redis
Graphite
Prometheus
pgprometheus
OpenMetrics Standard Proposal
Timescale Parallel Copy
Hadoop
PostGIS
KDB+
DevOps
Internet of Things
MongoDB
Elastic
DataBricks
Apache Spark
Confluent
New Enterprise Associates
MapD
Benchmark Ventures
Hortonworks
2σ Ventures
CockroachDB
Cloudflare
EMC
Timescale Blog: Why SQL is beating NoSQL, and what this means for the future of data
The intro and outro music is from The Hug by The Freak Fandango Orchestra / CC BY-SA
Summary
As communications between machines become more commonplace the need to store the generated data in a time-oriented manner increases. The market for timeseries data stores has many contenders, but they are not all built to solve the same problems or to scale in the same manner. In this episode the founders of TimescaleDB, Ajay Kulkarni and Mike Freedman, discuss how Timescale was started, the problems that it solves, and how it works under the covers. They also explain how you can start using it in your infrastructure and their plans for the future.
Preamble
Hello and welcome to the Data Engineering Podcast, the show about modern data infrastructure
When you’re ready to launch your next project you’ll need somewhere to deploy it. Check out Linode at dataengineeringpodcast.com/linode and get a $20 credit to try out their fast and reliable Linux virtual servers for running your data pipelines or trying out the tools you hear about on the show.
Go to dataengineeringpodcast.com to subscribe to the show, sign up for the newsletter, read the show notes, and get in touch.
You can help support the show by checking out the Patreon page which is linked from the site.
To help other people find the show you can leave a review on iTunes, or Google Play Music, and tell your friends and co-workers
Your host is Tobias Macey and today I’m interviewing Ajay Kulkarni and Mike Freedman about Timescale DB, a scalable timeseries database built on top of PostGreSQL
Interview
Introduction
How did you get involved in the area of data management?
Can you start by explaining what Timescale is and how the project got started?
The landscape of time series databases is extensive and oftentimes difficult to navigate. How do you view your position in that market and what makes Timescale stand out from the other options?
In your blog post that explains the design decisions for how Timescale is implemented you call out the fact that the inserted data is largely append only which simplifies the index management. How does Timescale handle out of order timestamps, such as from infrequently connected sensors or mobile devices?
How is Timescale implemented and how has the internal architecture evolved since you first started working on it?
What impact has the 10.0 release of PostGreSQL had on the design of the project?
Is timescale compatible with systems such as Amazon RDS or Google Cloud SQL?
For someone who wants to start using Timescale what is involved in deploying and maintaining it?
What are the axes for scaling Timescale and what are the points where that scalability breaks down?
Are you aware of anyone who has deployed it on top of Citus for scaling horizontally across instances?
What has been the most challenging aspect of building and marketing Timescale?
When is Timescale the wrong tool to use for time series data?
One of the use cases that you call out on your website is for systems metrics and monitoring. How does Timescale fit into that ecosystem and can it be used along with tools such as Graphite or Prometheus?
What are some of the most interesting uses of Timescale that you have seen?
Which came first, Timescale the business or Timescale the database, and what is your strategy for ensuring that the open source project and the company around it both maintain their health?
What features or improvements do you have planned for future releases of Timescale?
Contact Info
Ajay
LinkedIn
@acoustik on Twitter
Timescale Blog
Mike
Website
LinkedIn
@michaelfreedman on Twitter
Timescale Blog
Timescale
Website
@timescaledb on Twitter
GitHub
Parting Question
From your perspective, what is the biggest gap in the tooling or technology for data management today?
Links
Timescale
PostGreSQL
Citus
Timescale Design Blog Post
MIT
NYU
Stanford
SDN
Princeton
Machine Data
Timeseries Data
List of Timeseries Databases
NoSQL
Online Transaction Processing (OLTP)
Object Relational Mapper (ORM)
Grafana
Tableau
Kafka
When Boring Is Awesome
PostGreSQL
RDS
Google Cloud SQL
Azure DB
Docker
Continuous Aggregates
Streaming Replication
PGPool II
Kubernetes
Docker Swarm
Citus Data
Website
Data Engineering Podcast Interview
Database Indexing
B-Tree Index
GIN Index
GIST Index
STE Energy
Redis
Graphite
Prometheus
pg_prometheus
OpenMetrics Standard Proposal
Timescale Parallel Copy
Hadoop
PostGIS
KDB+
DevOps
Internet of Things
MongoDB
Elastic
DataBricks
Apache Spark
Confluent
New Enterprise Associates
MapD
Benchmark Ventures
Hortonworks
2σ Ventures
CockroachDB
Cloudflare
EMC
Timescale Blog: Why SQL is beating NoSQL, and what this means for the future of data
The intro and outro music is from The Hug by The Freak Fandango Orchestra / CC BY-SA