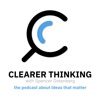
How quickly is AI advancing? And should you be working in the field? (with Danny Hernandez)
Clearer Thinking with Spencer Greenberg
English - August 23, 2023 23:59 - 1 hour - 63 MB - ★★★★★ - 119 ratingsSocial Sciences Science Homepage Download Apple Podcasts Google Podcasts Overcast Castro Pocket Casts RSS feed
Read the full transcript here.
Along what axes and at what rates is the AI industry growing? What algorithmic developments have yielded the greatest efficiency boosts? When, if ever, will we hit the upper limits of the amount of computing power, data, money, etc., we can throw at AI development? Why do some people seemingly become fixated on particular tasks that particular AI models can't perform and draw the conclusion that AIs are still pretty dumb and won't be taking our jobs any time soon? What kinds of tasks are more or less easily automatable? Should more people work on AI? What does it mean to "take ownership" of our friendships? What sorts of thinking patterns employed by AI engineers can be beneficial in other areas of life? How can we make better decisions, especially about large things like careers and relationships?
Danny Hernandez was an early AI researcher at OpenAI and Anthropic. He's best known for measuring macro progress in AI. For example, he helped show that the compute of the largest training runs was growing at 10x per year between 2012 and 2017. He also helped show an algorithmic equivalent of Moore's Law that was faster, and he's done work on scaling laws and mechanistic interpretability of learning from repeated data. He is currently focused on alignment research.
Staff
Spencer Greenberg — Host / DirectorJosh Castle — ProducerRyan Kessler — Audio EngineerUri Bram — FactotumWeAmplify — TranscriptionistsMiles Kestran — MarketingMusic
Broke for FreeJosh WoodwardLee RosevereQuiet Music for Tiny Robotswowamusiczapsplat.comAffiliates
Clearer ThinkingGuidedTrackMind EasePositlyUpLift[Read more]