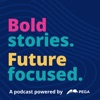
How can synthetic data change the world? ft. Phil Bayer, Beatrice Milik, Emna Amor, and Peter van der Putten
Bold stories. Future focused.
English - November 30, 2021 08:00 - 22 minutes - 50.7 MB - ★★★★★ - 11 ratingsManagement Business Technology pega businessinnovation digitalusecases futureofwork techtrends Homepage Download Apple Podcasts Google Podcasts Overcast Castro Pocket Casts RSS feed
As AI becomes more capable, it must collect more data to adapt better to our world. By creating data artificially (and not at the expense of our privacy), our future becomes smarter that much faster. From teaching autonomous cars how to drive using 3D content to helping the blind run without fear using machine learning, our guests talk share the ways they've used synthetic data to achieve big things – and talk about even greater things it can make possible.
Key Takeaways:
[2:01] Beatrice and her fellow researchers were handing over their own data to fill gaps in the studies when they did not have enough data, or when there were challenges around convincing subjects to be part of their study. So how could synthetic data have solved these problems?
[2:54] Synthetic data is data that is artificially created and has the same statistical properties as the original data. However, when you generate synthetic data, the process is completely irreversible.
[3:50] Without synthetic data, if the world has biased information, you may not see equal representation of people in all places and data that represents them. Phil Bayer, an engineer at Google’s Project Guideline, discusses how data bias is no small concern.
[6:17] Emna talks about how AI needs to develop new algorithms and methods to detect things and to mimic human behavior, with self-driving cars as an example.
[9:07] Phil and his team at Project Guideline are working on a project that allows blind individuals to run outside by detecting a yellow line on the ground. Phil talks about how it all began when a man named Thomas Panek walked into a Google Hackathon and asked if they could help blind people run freely. A few surreal data sets later, Phil was moved by watching Thomas run freely outside.
[14:36] Robotic systems might benefit from some virtual reality training, and using 3D environments to train AI on synthetic data is just the tip of the iceberg when we look at what is possible. Peter van der Putten, Director of Decisioning and AI Solutions at Pegasystems, and Prof of Media Technology at Leiden University speaks about how a lot of VR is too perfect, and we can benefit when it has the graininess and character of the real world.
[16:57] After Grand Theft Auto 5 was released in 2013, Intel researchers decided to try to make a movie version of the game that would be more photorealistic. They used a machine learning technique that used real-world data.
[20:02] Peter’s student research has some interesting implications for how AI systems train best in virtual reality. Phil agrees, saying that using VR as a training site for AI just might be the way of the future.
Quotes:
“We basically, as consumers or customers of any type, have no privacy at all. So, of course, I wanted to join this mission to build a technology that would eventually give us what is ours back.” - Beatrice 3:15 “Synthetic data can even help us balance out some of the biases we see in the real world. With synthetic data, you can create worlds that sort of you are hoping for, or that you're envisioning.” - Phil 5:12 “With synthetic data, you can create realistic 3D content and without too much human effort and you can make more areas diverse.” - Emna 7:09 “To see sort of the variety of ways in which someone can be helped by technology — like this is really powerful.” - Phil 14:11 “A lot of VR is hyper-realistic. It's not that it's not perfect enough. It's too perfect. It's missing the graininess, and the glossy character of the real world.” - Peter 15:58 “The other thing that has been showing a lot of promise in synthetic data is helping to try and remove bias from datasets. And so I think that's another reason why it's growing in popularity.” Phil - 19:40 “We can create less biased AI. We can share our data with confidence in our privacy.” James - 20:31
Continue on your journey:
Mentioned:
Grand Theft Auto Article by the Imperial College London Beatrice Milik Emna Amor Philip Bayer Peter van der Putten